Comparison of Statistical and Machine-Learning Models on Road Traffic Accident Severity Classification by Infante et al. (2022)
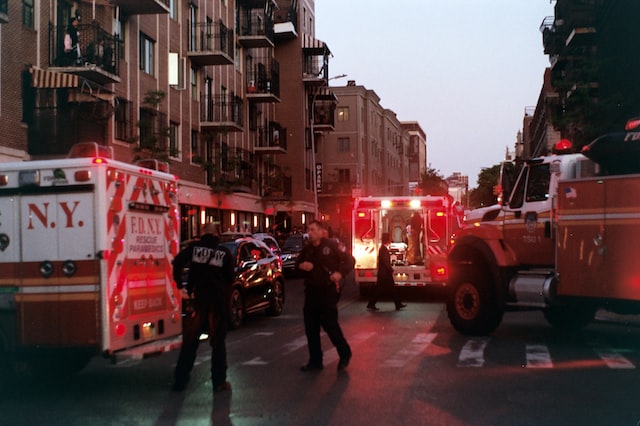
The authors concluded that machine-learning methods are not well-suited for the most serious road traffic accident datasets due to the large number of training observations they require. The significance of this finding has been previously discussed. The machine-learning models performed exceptionally well when the dataset contained a bigger sample of the class with the highest severity as well as when the dataset was more evenly distributed. In spite of this, the statistical logistic regression model was able to achieve comparable performance with the added benefit of knowing more about the significance of the variables in explaining the risk factors. It is our intention to replicate and expand this research onto other datasets to provide further evidence for and generalizability of our findings. To further validate the results of this set of experiences, we want to apply this methodology to data from other regions in Portugal. In further research, we hope to investigate the effects of using varying training and testing dataset sizes, as well as various methods for taking the lead with skewed data. In particular, we want to investigate the feasibility of using machine-learning techniques for the detection of extremely uncommon occurrences. The use of neural network architectures, and more specifically deep-learning approaches, to spot anomalies in time-series data is another goal of ours, and we plan to put them to the test in this work.