Predicting multiple types of traffic accident severity with explanations: A multi-task deep learning framework by Yang et al. (2022)
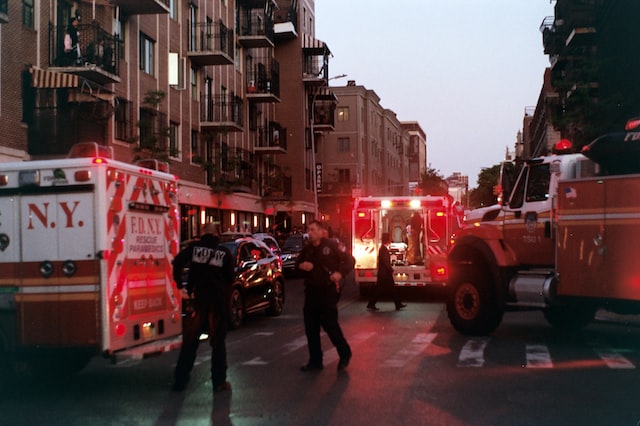
Predicting the severity of a traffic collision is important for a number of reasons, including avoiding similar incidents in the future and reducing the impact of accidents that have already occurred. In this research, we develop a multi-task deep learning framework that can predict the severity of injuries, fatalities, and property damage. Key contributors to the three levels of traffic accident severity are also explained by our model. To the best of our knowledge, our model is the first multitask learning model and the first DNN-based model for predicting the severity of traffic accidents. Here are some of our study’s most significant findings and contributions. To begin, our framework’s multi-task and deep learning design not only gives professionals access to a thorough analysis of traffic accident severity but also improves the accuracy of predictions. Our model outperforms state-of-the-art methods when compared to other baseline models using data on road accidents in China. Second, our model’s LRP explanations aid in pinpointing important aspects that contribute to the severity of traffic accidents. Discrete outcome models, data mining approaches, and other soft computing models are compared to our suggested model, which is shown to provide the best explanation quality through a series of case studies. Thirdly, the proposed multi-task deep learning framework yields valuable insights that can help researchers and practitioners in the field of VRU safety. Our suggested multi-task deep learning architecture has real-world applications, such as assisting in the creation of VRU safety policies and facilitating smart city development. As a result of our suggested model’s accurate prediction and identified important elements, transportation and urban development agencies may take steps to limit the number of traffic incidents that result in personal injury, wrongful death, or damage to public or private property. Government transportation agencies could use our model and its explanations to improve their capacity to foresee and respond to traffic accidents, identify the root causes of the most serious ones, select appropriate safety countermeasures and policies, and resolve problems in transportation and urban development. In Subsection 4.3, for instance, a case study demonstrates how the severity of injuries and fatalities in traffic accidents is influenced by the state of the roads. This data might be used by city maintenance crews to keep tabs on the state of the roads and reduce the number of people killed or injured in traffic incidents. Safety regulations, initiatives, and plans like these from the government not only make streets safer for pedestrians and drivers, but also help push forward the concept of “smart cities” (Anisetti et al., 2018; Chehri et al., 2020). Many avenues could be pursued in future studies. As a first step, we will make use of more enhanced data if we can find suitable data sources. It would be possible, for instance, to supplement the data with images of the automobile and the route. Second, our model might be more easily explained to others. Methods for obtaining decision rules from DNNs may be developed in the future. Third, our model might be improved by incorporating more complex multi-task methodologies, which would increase collaboration across the three jobs of predicting traffic accident severity and deliver state-of-the-art protection measures.